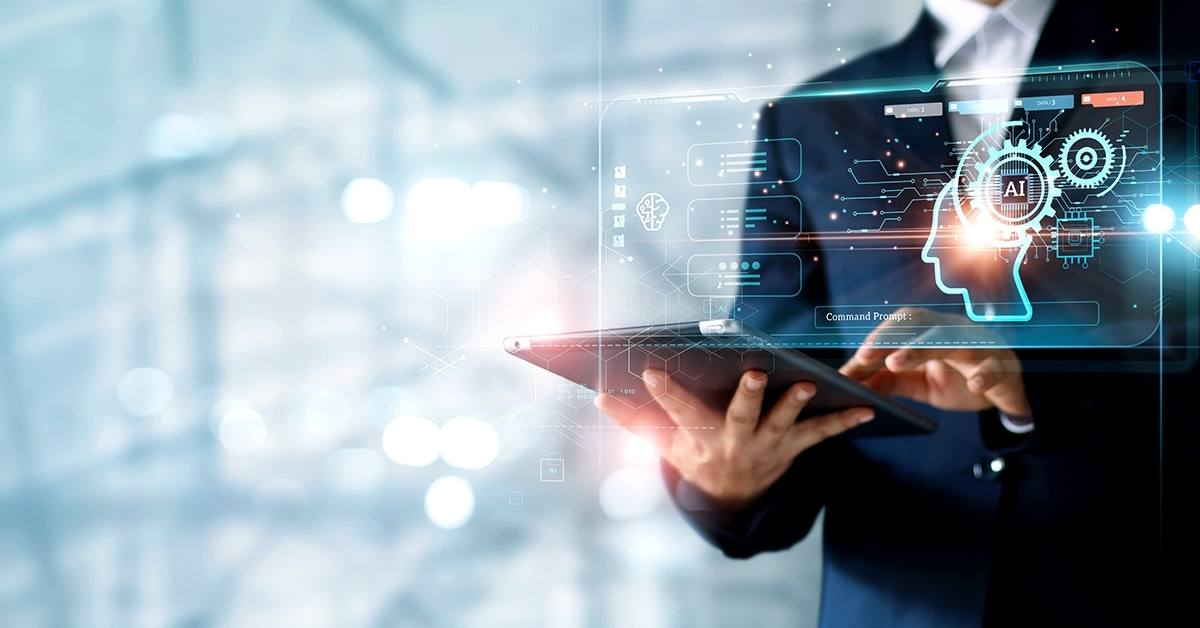
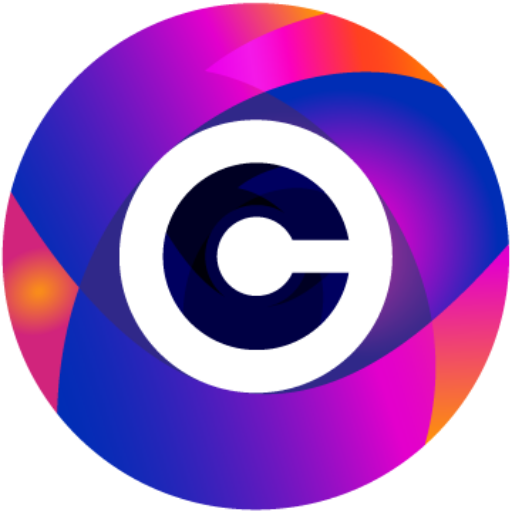
Greg Druffel • Managing Solution Architect
Can you think of any truly utopian sci-fi movies about Artificial Intelligence (AI)? Even lighter-hearted ones can’t resist the sci-fi trope of AI going rogue. A recently released film, The Creator, is a beautifully shot example of the typical dystopian AI tale — likely hoping to capitalize on society’s recent stress over the dramatic advances in AI.
So, is our future with AI destined to be all doom and gloom like the movies? Definitely not. This blog is the second in a five-part series discussing one of many applications of AI that register much closer to the utopian end of the scale, Artificial Intelligence for IT operations, or AIOps.
As we discussed in our first blog in this series, AIOps helps companies ingest and analyze the overwhelming quantity of data and alerts generated by IT operations to identify patterns, trends, and anomalies. It learns from these and then makes predictions, initiates automated remediations, or offers recommendations that will aid IT teams in decision-making. The end goals are boosted efficiency, less downtime, and cost savings.
And who wouldn’t want to achieve those? But implementing AIOps is not as simple as buying a platform like Moogsoft or Splunk.
The key to a successful implementation is integrating the right toolsets with your infrastructure to enable three main functionalities:
- Data ingestion (from multiple sources across the infrastructure)
- Analysis, correlation, and recommendations
- Proactive remediation (the fix)
In this blog, we’ll look more closely at the first two parts of the process: ingestion of the data and the subsequent analysis, correlation, and generation of recommendations. Part two will focus on how AIOps fixes issues and enables proactive remediation.
A Closer Look at Data Ingestion
In an enterprise organization, the applications, infrastructure, and network are often siloed, perhaps even delivered by multiple providers. This makes it challenging to gain a comprehensive overview of all IT domains to best manage them.
To complicate issues further, each component of IT operations may come with its own “language” or terminology for attributes. An effective AIOps framework creates a common syntax of attributes and data classes as it collects and integrates real-time and historical data from across a company’s various sources to build greater visibility and allow for cross-domain analysis of issues.
The sources could be different hosting models, such as on-premises, private cloud, public cloud, or hybrid. The systems needing monitoring might include:
- Point of Sale (PoS),
- Internet of Things (IoT) devices,
- PCs, servers, computers,
- Infrastructure, applications, middleware, databases, and backup.
Collecting the data for AIOps may mean plugging in existing monitoring tools, ticketing systems, and incident management systems, leveraging performance monitoring from service providers, or instrumenting the environment with monitoring tools.
Whatever the origin of data collected, it’s vital that the AIOps framework can handle the volume and scale as the organization grows.
Taming the Data, Identifying Incidents, and Finding the Root Cause
Event Analysis
Once the data is ingested, the AIOps platform applies machine learning algorithms to filter, remove duplicates, normalize, and correlate events across multiple siloes, boiling the data down into more manageable “incidents.”
The algorithms used in incident analysis continuously improve over time in two ways: learning from results applied to previous incidents and through manual training by operators.
Incident Enrichment and Management
Alerts and incidents can be enriched with data and details from external sources such as ITSM tools, financial systems, and business databases to aid in the diagnosis and remediation process.
Diagnosis and Recommendations
The AIOps platform runs diagnostics to identify the root cause. For example, it may run an automation to collect more information from an endpoint. It then determines how an incident can be resolved and builds recommendations.
If the diagnostics cannot determine a resolution, AIOps escalates the incident and may dispatch a technician to perform further diagnostics.
Get a Handle on Your IT Operations
In this blog, we’ve delved deeper into how AIOps can help you cut through the noise of overwhelming amounts of data and alerts to get to the crux of issues in IT operations, whether it be a predicted slowdown or anomalies indicating a security threat. Our next blog will focus on the final step in a more sophisticated AIOps implementation: proactive remediation.
Later, we’ll finish our series by showing how an experienced provider such as Compucom can help you go beyond the buzzword to a truly effective implementation and hear some real-world success stories.
In this series:
Recent Posts
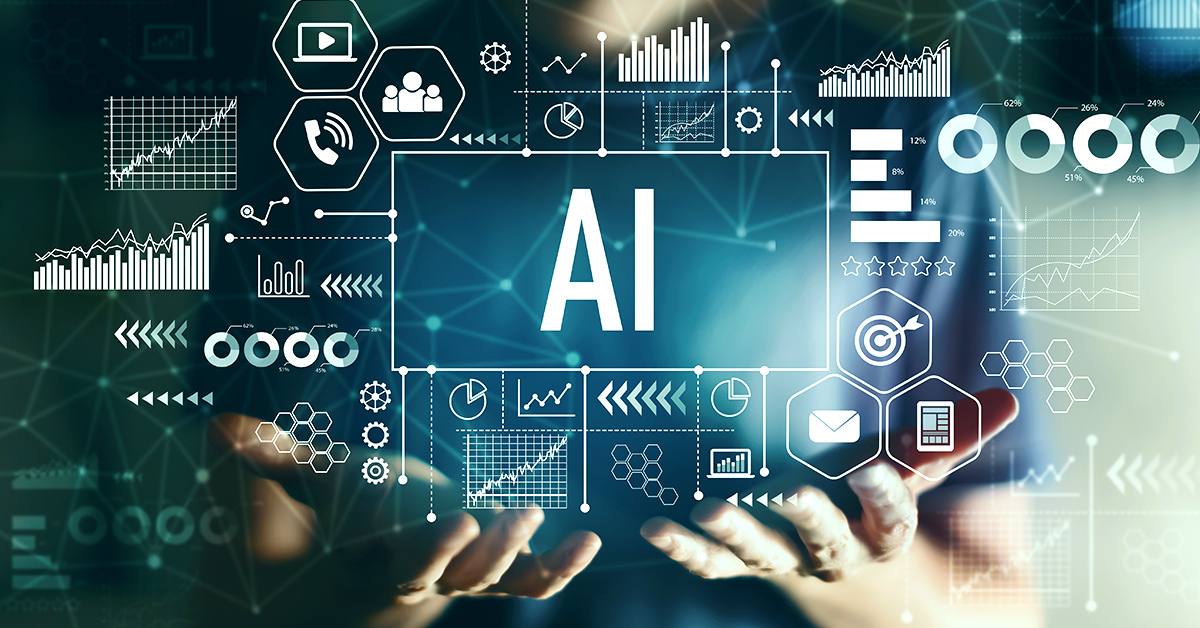
Real-World AIOps: Examples and Benefits
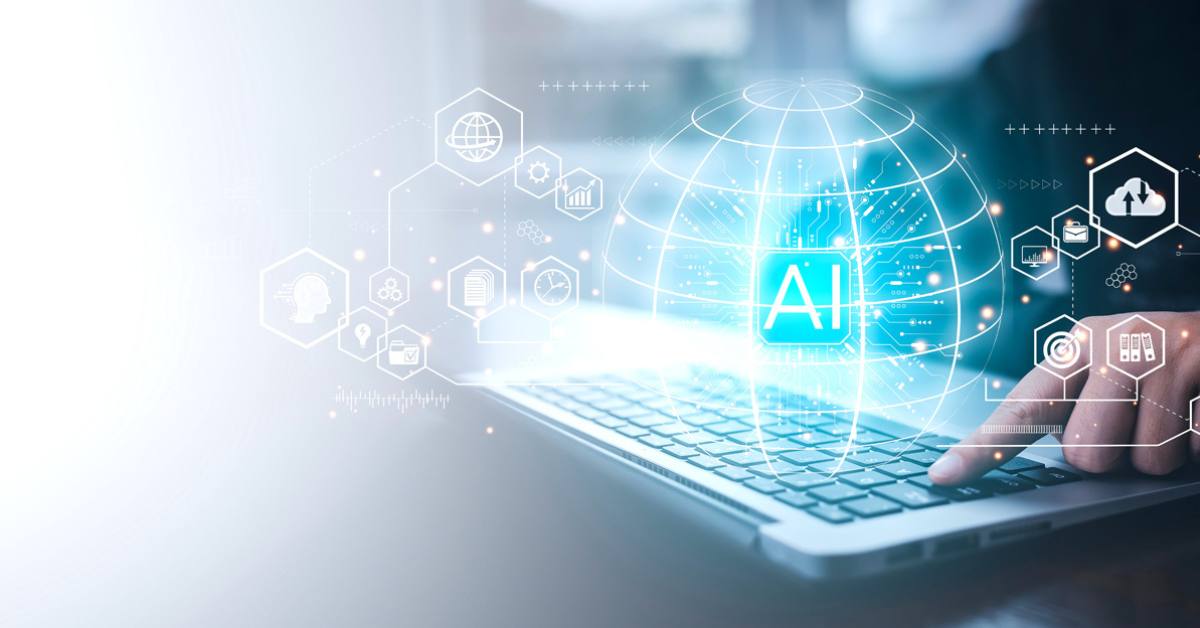
AIOps: Going Beyond the Buzzword
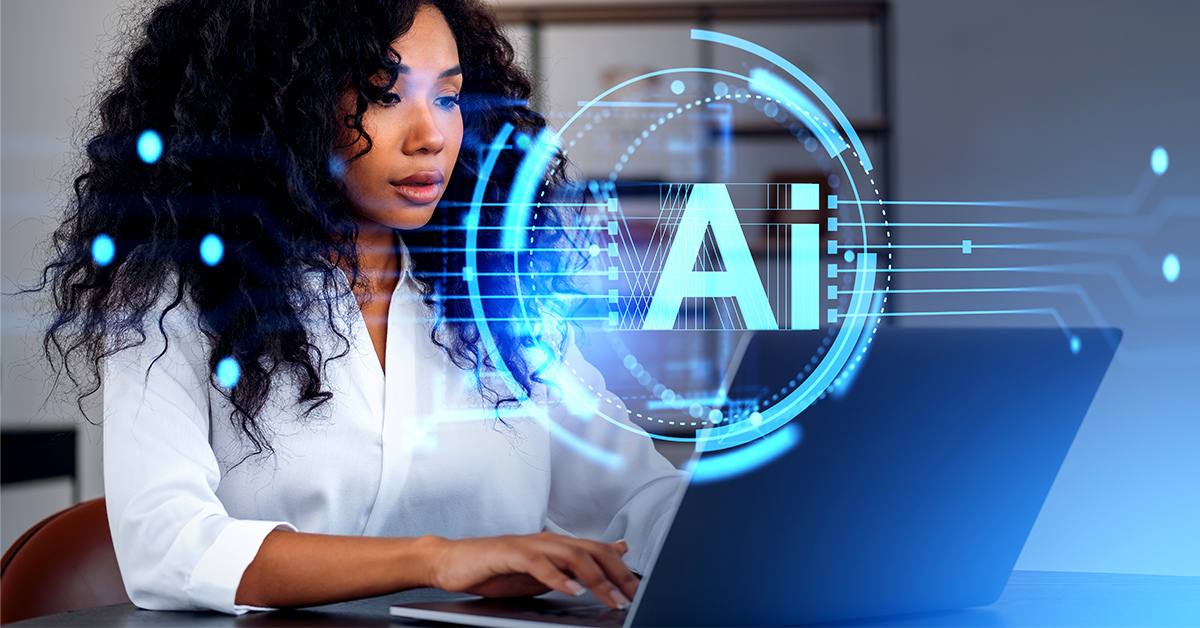
How AIOps Works: Continually Smarter & More Effective IT Operations
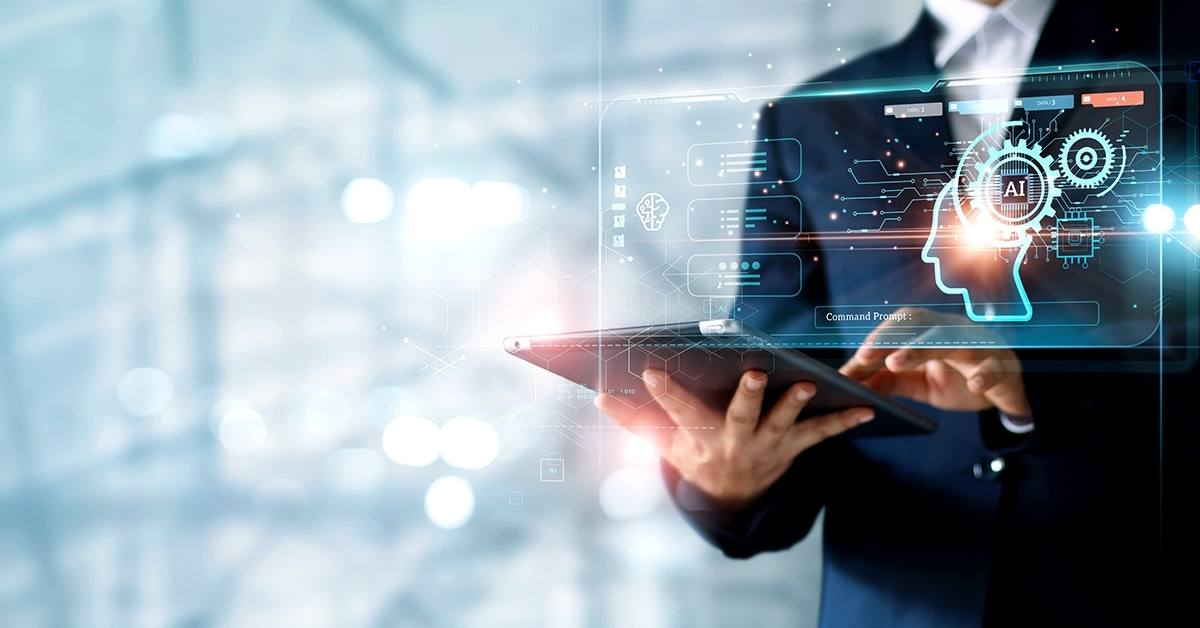
How AIOps Works: Tame Big Data and Get to the Crux of the Matter
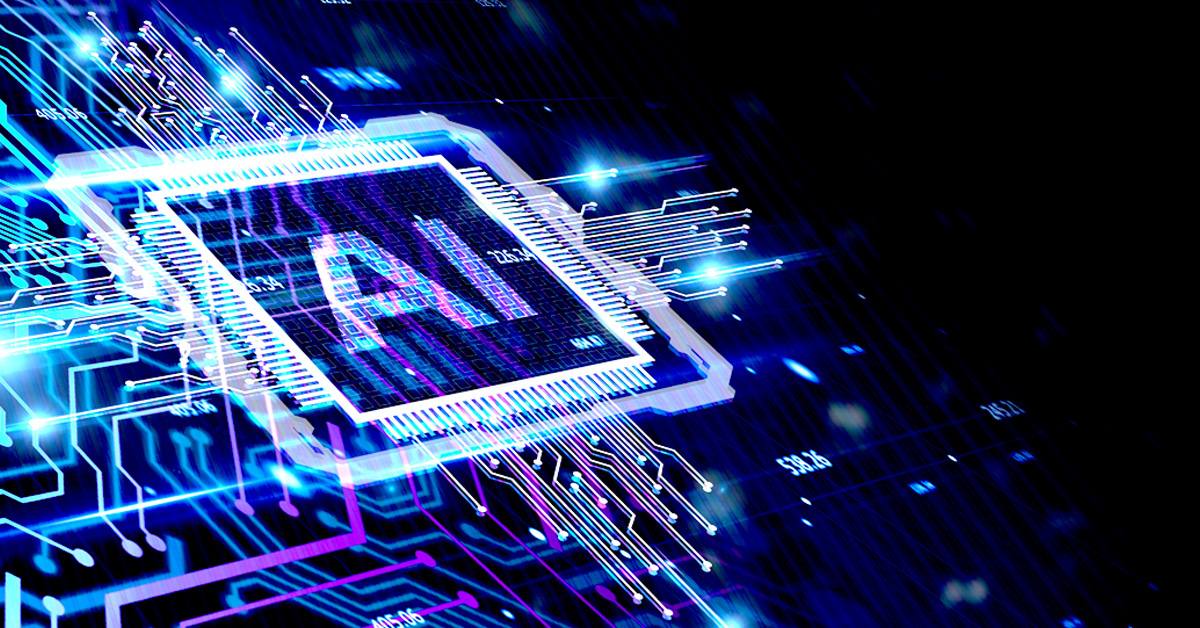
The Big Deal About AIOps
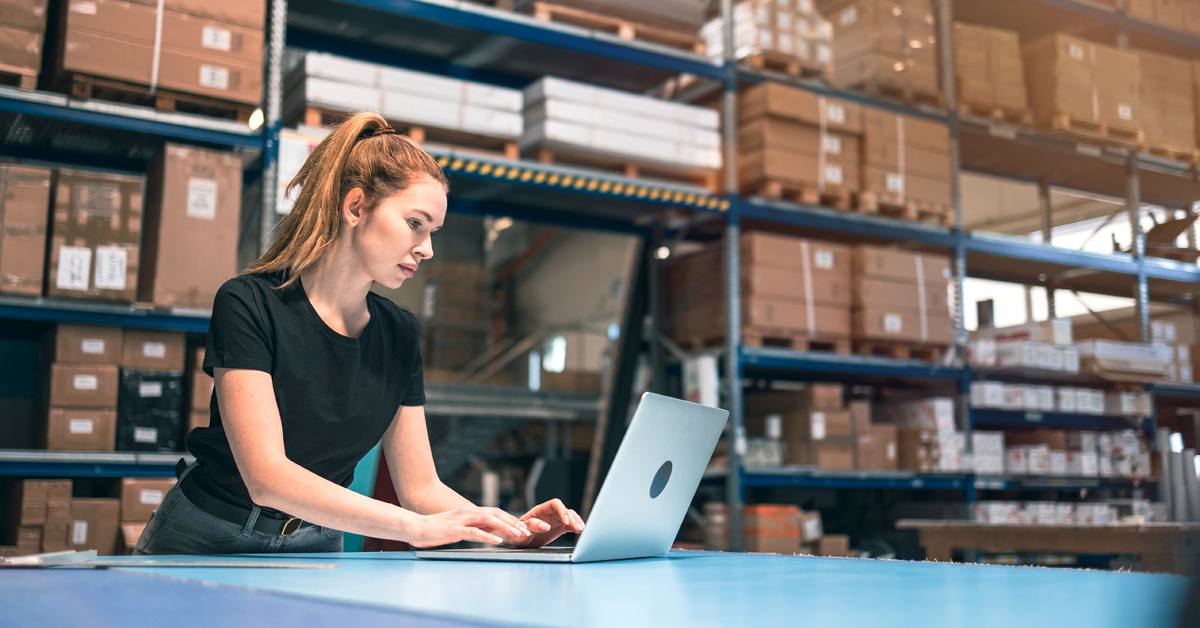
There’s More to IT Hardware Procurement Than Getting the Best Deal
CATEGORIES
How AIOps Works: Tame Big Data and Get to the Crux of the Matter
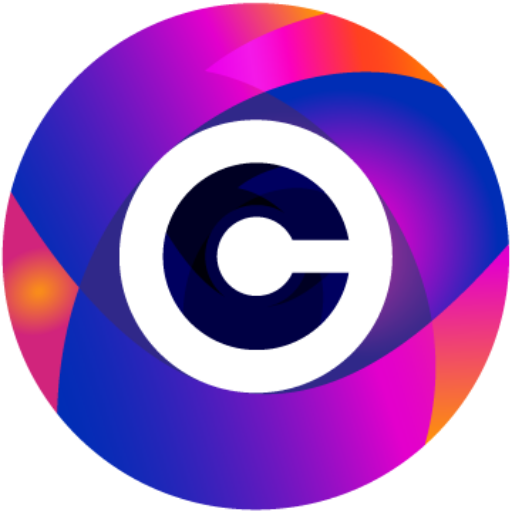
Greg Druffel • Managing Solution Architect
Can you think of any truly utopian sci-fi movies about Artificial Intelligence (AI)? Even lighter-hearted ones can’t resist the sci-fi trope of AI going rogue. A recently released film, The Creator, is a beautifully shot example of the typical dystopian AI tale — likely hoping to capitalize on society’s recent stress over the dramatic advances in AI.
So, is our future with AI destined to be all doom and gloom like the movies? Definitely not. This blog is the second in a five-part series discussing one of many applications of AI that register much closer to the utopian end of the scale, Artificial Intelligence for IT operations, or AIOps.
As we discussed in our first blog in this series, AIOps helps companies ingest and analyze the overwhelming quantity of data and alerts generated by IT operations to identify patterns, trends, and anomalies. It learns from these and then makes predictions, initiates automated remediations, or offers recommendations that will aid IT teams in decision-making. The end goals are boosted efficiency, less downtime, and cost savings.
And who wouldn’t want to achieve those? But implementing AIOps is not as simple as buying a platform like Moogsoft or Splunk.
The key to a successful implementation is integrating the right toolsets with your infrastructure to enable three main functionalities:
- Data ingestion (from multiple sources across the infrastructure)
- Analysis, correlation, and recommendations
- Proactive remediation (the fix)
In this blog, we’ll look more closely at the first two parts of the process: ingestion of the data and the subsequent analysis, correlation, and generation of recommendations. Part two will focus on how AIOps fixes issues and enables proactive remediation.
A Closer Look at Data Ingestion
In an enterprise organization, the applications, infrastructure, and network are often siloed, perhaps even delivered by multiple providers. This makes it challenging to gain a comprehensive overview of all IT domains to best manage them.
To complicate issues further, each component of IT operations may come with its own “language” or terminology for attributes. An effective AIOps framework creates a common syntax of attributes and data classes as it collects and integrates real-time and historical data from across a company’s various sources to build greater visibility and allow for cross-domain analysis of issues.
The sources could be different hosting models, such as on-premises, private cloud, public cloud, or hybrid. The systems needing monitoring might include:
- Point of Sale (PoS),
- Internet of Things (IoT) devices,
- PCs, servers, computers,
- Infrastructure, applications, middleware, databases, and backup.
Collecting the data for AIOps may mean plugging in existing monitoring tools, ticketing systems, and incident management systems, leveraging performance monitoring from service providers, or instrumenting the environment with monitoring tools.
Whatever the origin of data collected, it’s vital that the AIOps framework can handle the volume and scale as the organization grows.
Taming the Data, Identifying Incidents, and Finding the Root Cause
Event Analysis
Once the data is ingested, the AIOps platform applies machine learning algorithms to filter, remove duplicates, normalize, and correlate events across multiple siloes, boiling the data down into more manageable “incidents.”
The algorithms used in incident analysis continuously improve over time in two ways: learning from results applied to previous incidents and through manual training by operators.
Incident Enrichment and Management
Alerts and incidents can be enriched with data and details from external sources such as ITSM tools, financial systems, and business databases to aid in the diagnosis and remediation process.
Diagnosis and Recommendations
The AIOps platform runs diagnostics to identify the root cause. For example, it may run an automation to collect more information from an endpoint. It then determines how an incident can be resolved and builds recommendations.
If the diagnostics cannot determine a resolution, AIOps escalates the incident and may dispatch a technician to perform further diagnostics.
Get a Handle on Your IT Operations
In this blog, we’ve delved deeper into how AIOps can help you cut through the noise of overwhelming amounts of data and alerts to get to the crux of issues in IT operations, whether it be a predicted slowdown or anomalies indicating a security threat. Our next blog will focus on the final step in a more sophisticated AIOps implementation: proactive remediation.
Later, we’ll finish our series by showing how an experienced provider such as Compucom can help you go beyond the buzzword to a truly effective implementation and hear some real-world success stories.
In this series:
Recent Posts
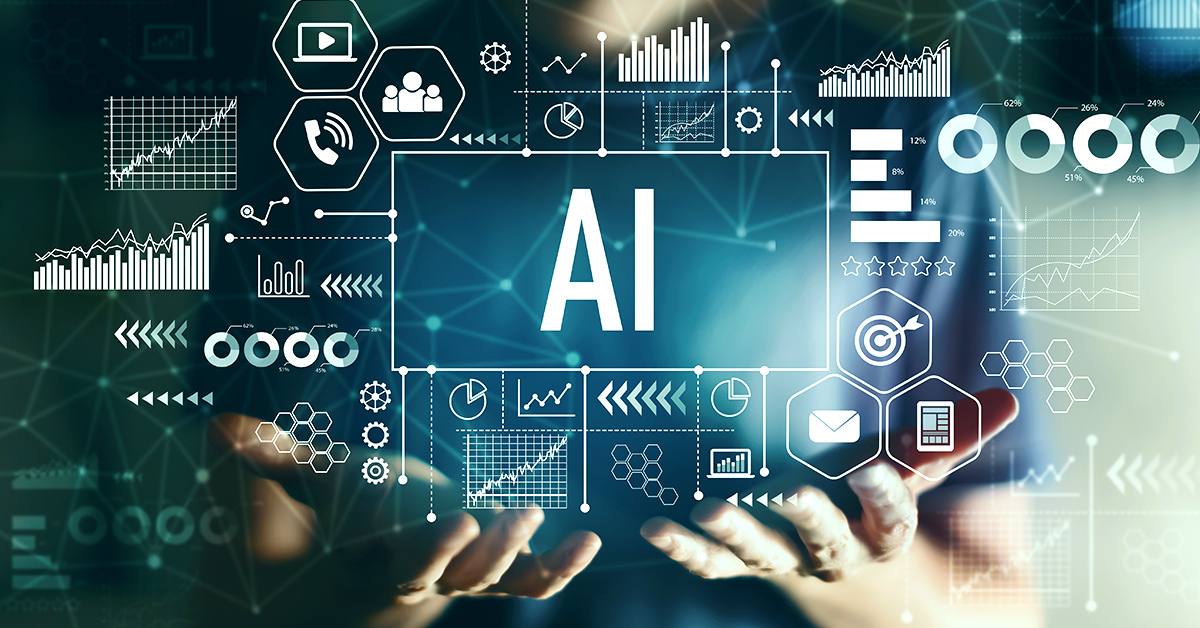
Real-World AIOps: Examples and Benefits
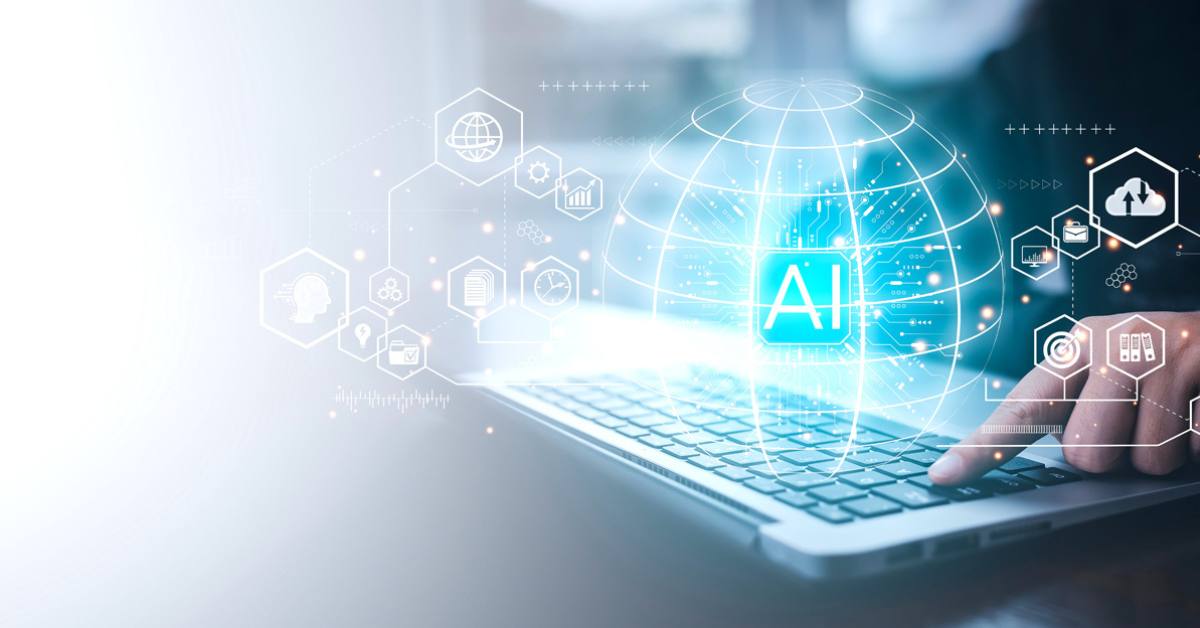
AIOps: Going Beyond the Buzzword
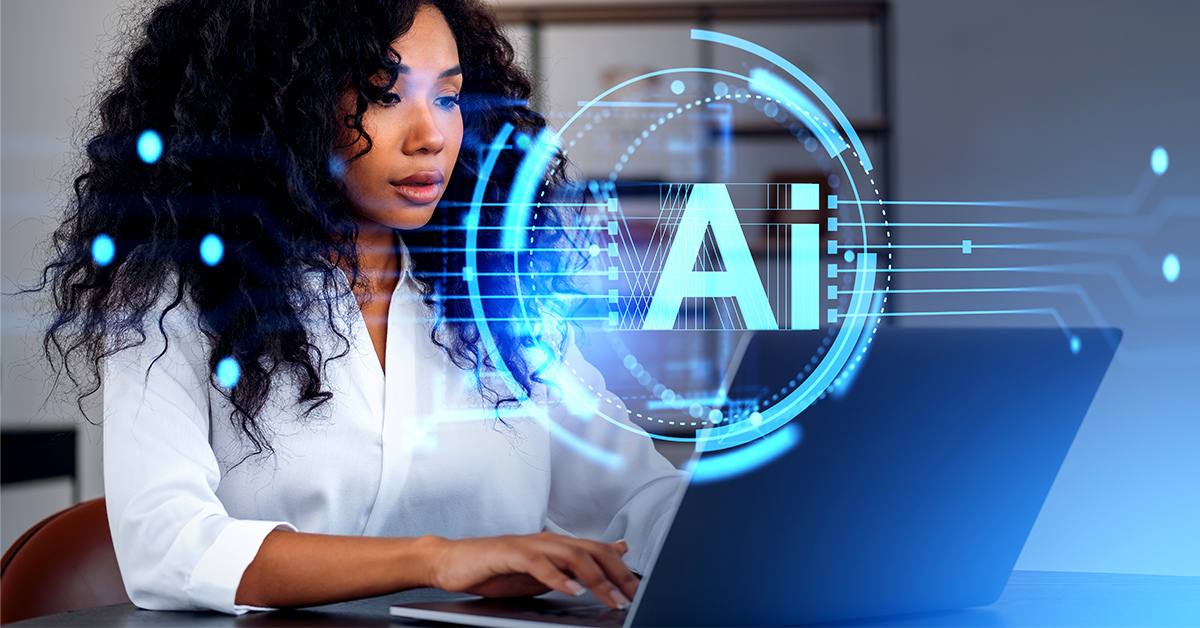
How AIOps Works: Continually Smarter & More Effective IT Operations
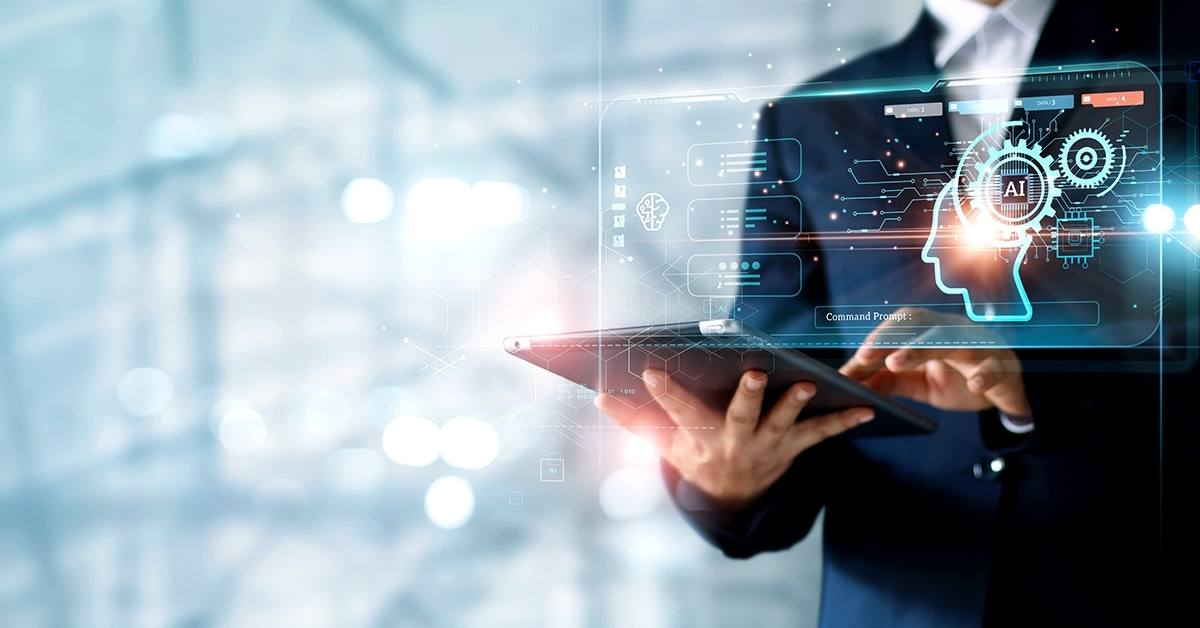
How AIOps Works: Tame Big Data and Get to the Crux of the Matter
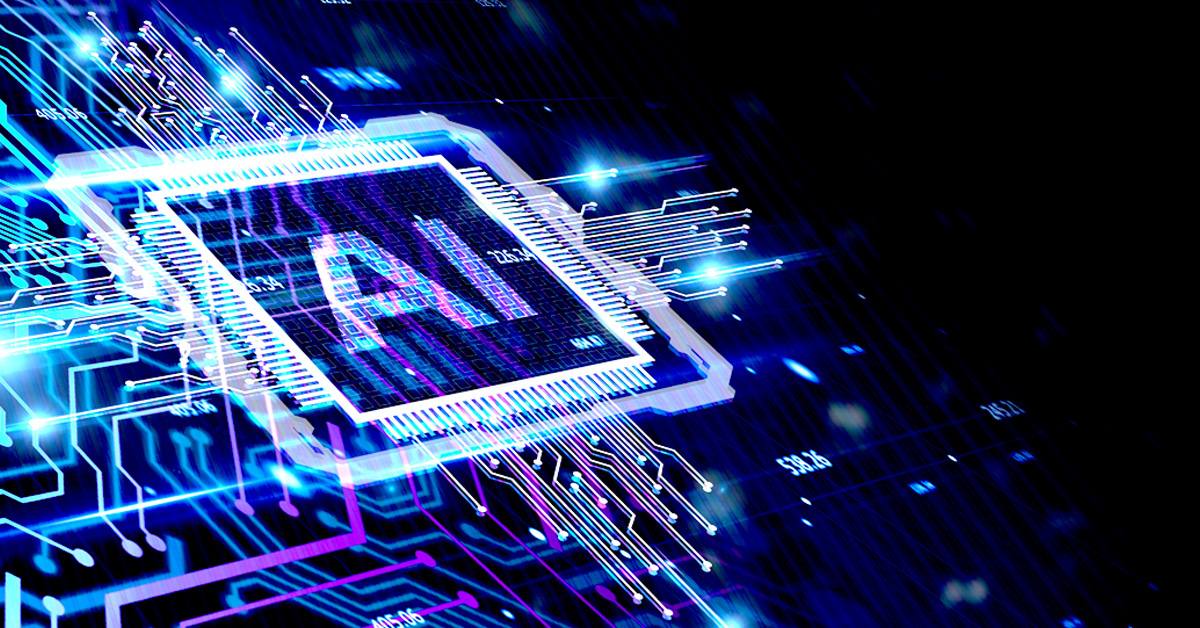
The Big Deal About AIOps
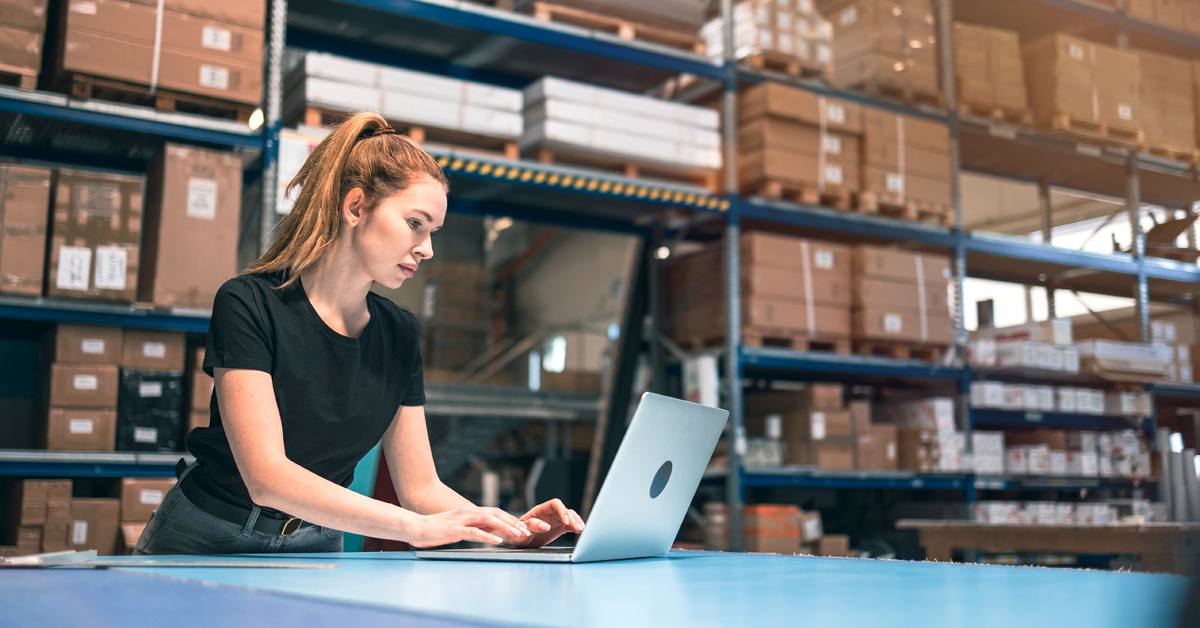
There’s More to IT Hardware Procurement Than Getting the Best Deal
CATEGORIES
Ready to Create an Innovative Workplace?
Recent Blog Posts
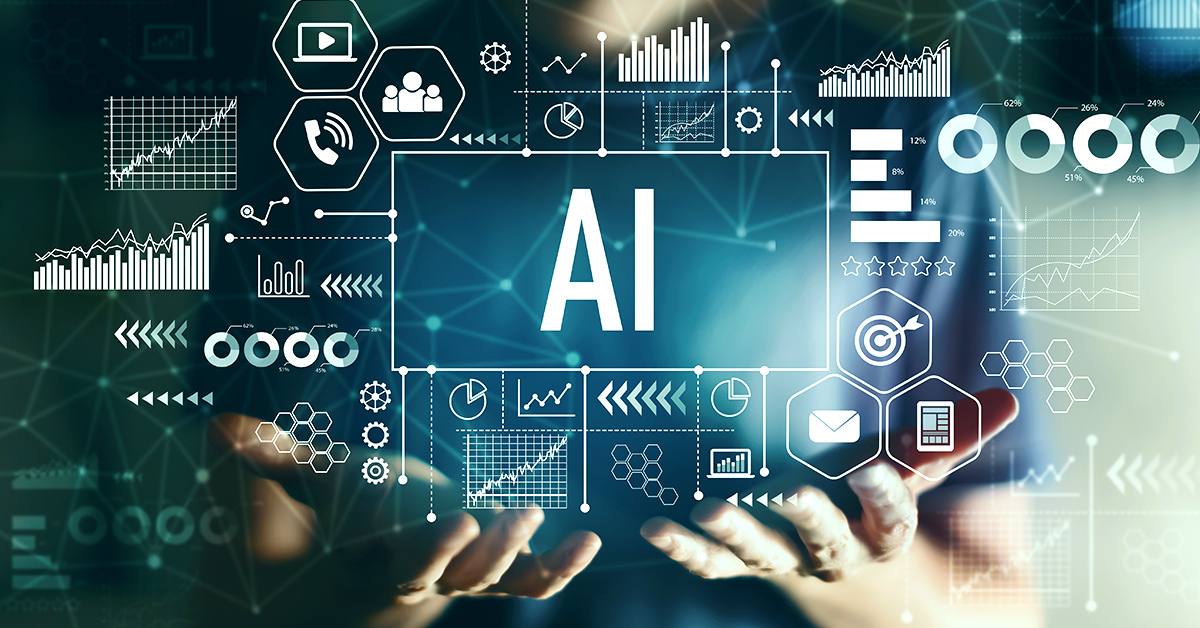
Real-World AIOps: Examples and Benefits
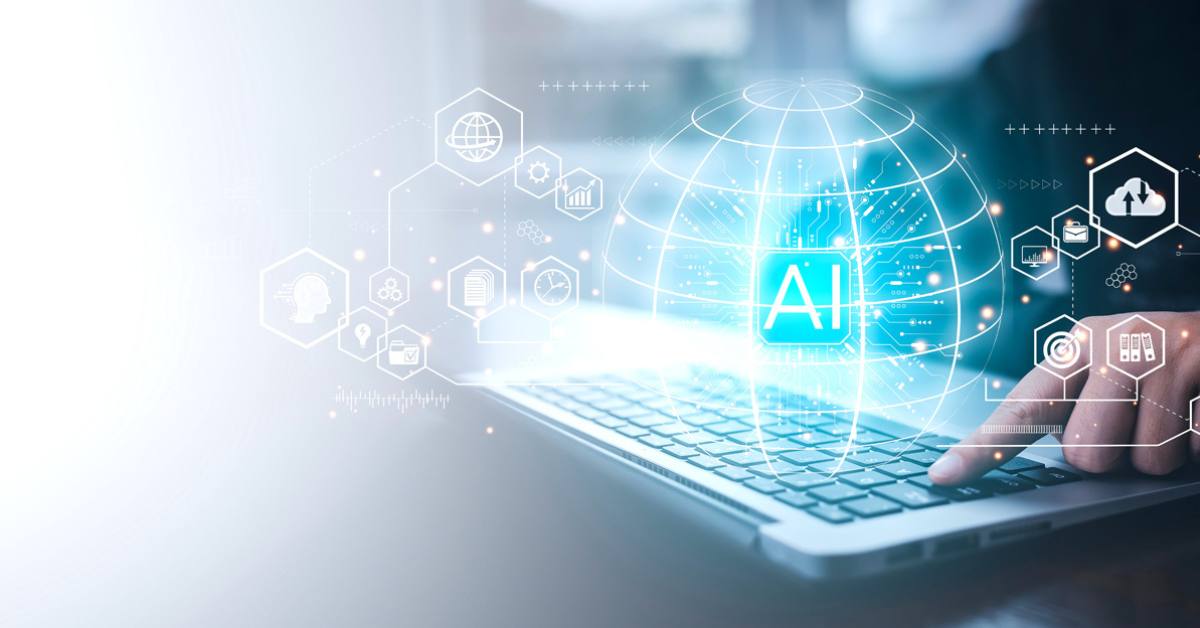
AIOps: Going Beyond the Buzzword
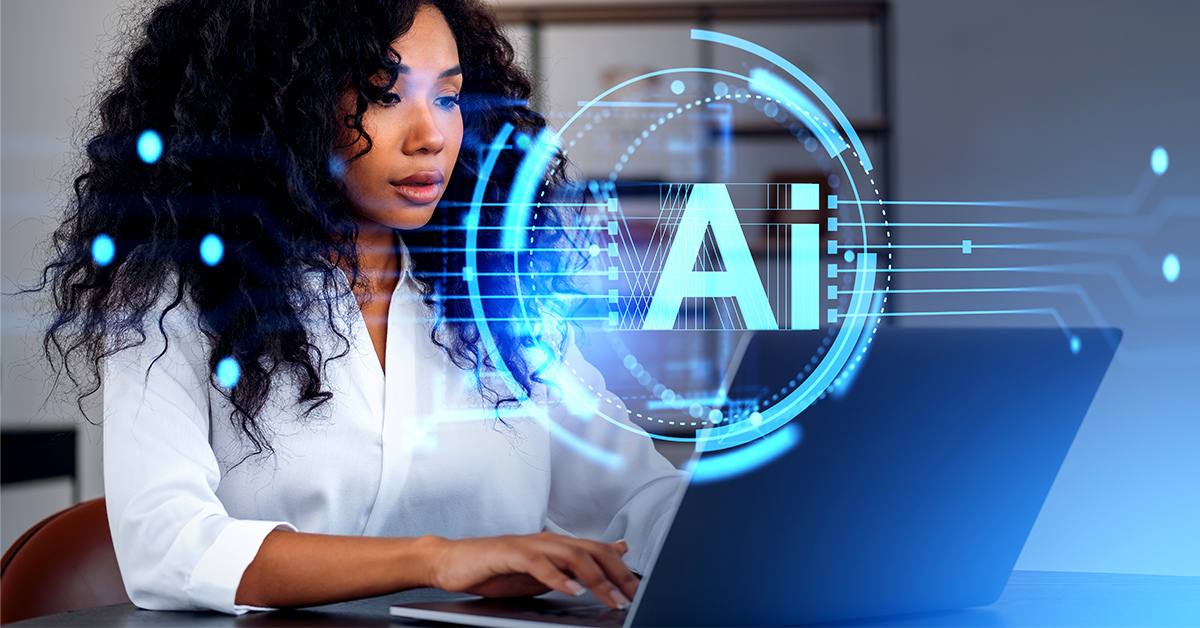