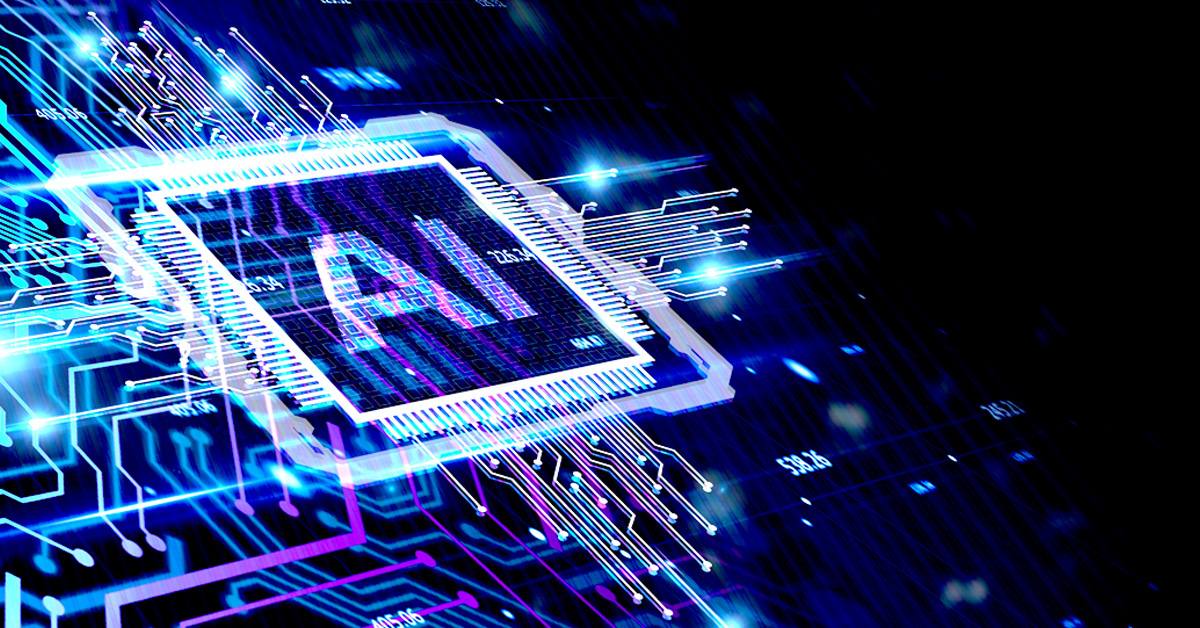
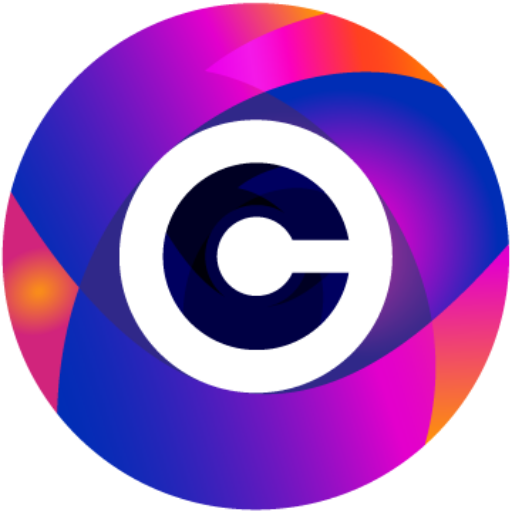
Greg Druffel • Managing Solution Architect
Why It’s the Future of IT Operations
How do you feel when you hear the term Artificial Intelligence (AI)? Excited? Anxious? Afraid? Suspicious? Were you an early adopter of ChatGPT, happily entering prompts to generate a week’s worth of Mediterranean diet recipes, or a blog that sounds like it Snoop Dogg or Cookie Monster wrote it? Or does the mention of AI make you shudder with thoughts of deep fakes and conspiracies, the voice of HAL 9000, SkyNet, and glitches in The Matrix? Frankly, for many of us, it’s a bit of both.
Whatever our trepidations, AI has rapidly moved from the realm of sci-fi to become part of our everyday lives. And the abundance of practical and beneficial applications for AI in business is undeniable – freeing us up from grunt work, augmenting our analytical capabilities, and saving us from costly human errors.
AIOps, or AI for IT Operations, is one such example. It uses Big Data, Machine Learning (ML), and AI to automate and improve how IT operations are managed, making them more efficient, reliable, and effective. This first blog in our five-part AIOps series gives an overview of AIOps and its benefits.
Cutting Through the Noise to Find and Fix the Issue
In enterprise organizations with complex and vast IT ecosystems, it’s becoming tougher to maintain a firm grip on IT operations. A typical enterprise organization has numerous applications, services, and infrastructure components in varied locations, generating huge amounts of data and requiring constant monitoring and analysis.
Pair this with increased user expectations and dependence on technology, and it’s easy to see the need for a system to help your IT teams cut through the noise to understand and predict which improvements are needed. And if this system can also automate some remedial actions, you’ll greatly augment your capability to keep your IT operations running smoothly and efficiently.
How Big Data and Machine Learning Work Together in AIOps
Big Data for AIOps refers to large, diverse sets of information sourced from monitoring systems and engagement data such as events, logs, performance, availability, and capacity.
ML is a subset of AI that focuses on algorithms that can learn from data and improve their performance without being explicitly programmed. In AIOps, ML can help discover patterns, trends, and correlations in the data, using them to make predictions and recommendations. It also enables AIOps to adapt to evolving environments and user needs by using feedback loops and reinforcement learning.
AIOps uses Big Data, AI, and ML capabilities to:
- Collect ever-increasing volumes of data from multiple IT infrastructure components.
- Intelligently pinpoint significant events and patterns related to performance and availability.
- Diagnose the root cause for automatic or human resolution.
The Components of an AIOps Implementation
According to a recent study, 67% of enterprise infrastructure is now cloud-based. A typical infrastructure can often be a combination of on-premises, private, and public cloud components and may be managed by multiple IT teams.
A successful AIOps implementation will carefully integrate several toolsets to help teams collaborate to observe, analyze, and act proactively to fix IT operations issues. These toolsets can be categorized into three functions:
- Data Ingestion
Observability tools handle the ingestion, aggregation, and analysis of performance data from your applications, infrastructure, and network. - Analysis, Correlation, and Recommendations
AIOps solutions analyze, correlate, and enrich the data for better insights and automated actions, enabling IT teams to filter out the noise from alerts and zero in on anomalies, patterns, and trends in resource requirements to get the big picture of your IT operations in real-time. - Proactive Remediation
AIOps tools can execute automated processes in response to recommendations, rectifying issues such as slowdowns promptly, sometimes before they even happen.
Get a Handle on Your IT Operations
Today’s companies face increased security threats and compliance requirements, difficulties managing vast quantities of system-generated data, and demands for complex and varied analysis by various stakeholders. Add to this list a shortage of IT talent to handle it all and it’s easy to see why there’s a dramatic rise in demand for AIOps.
As we continue with this blog series, we’ll dive deeper into how the components of AIOps work together, look at how an experienced provider like Compucom can help you go beyond the buzzword to realize its benefits and hear some real-world success stories.
In this series:
Recent Posts
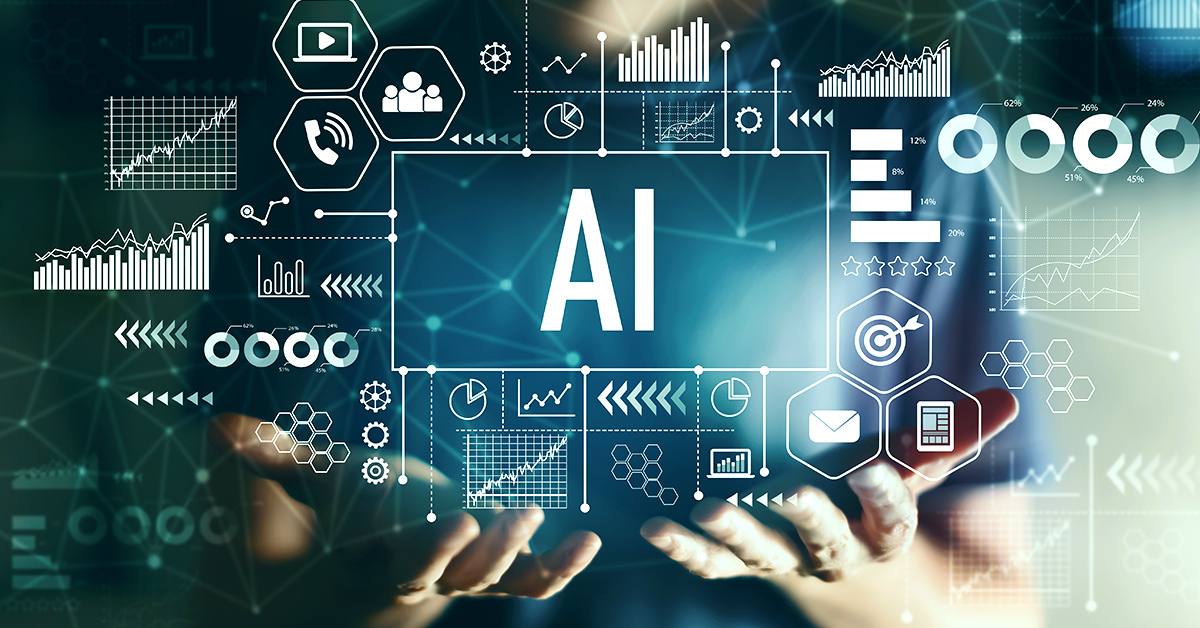
Real-World AIOps: Examples and Benefits
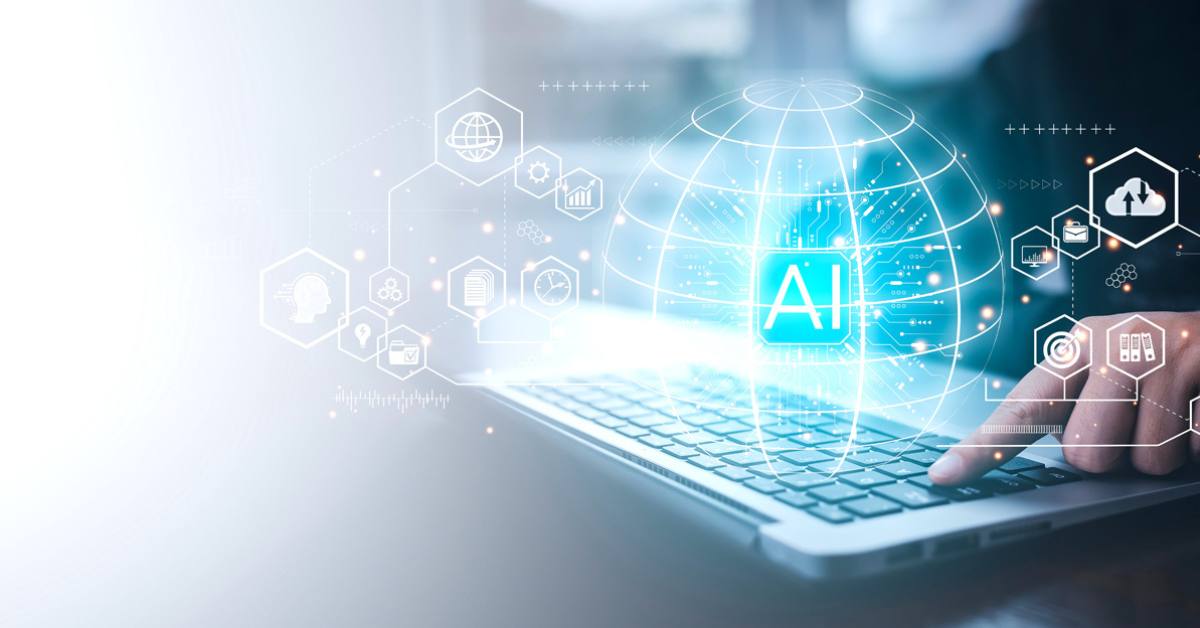
AIOps: Going Beyond the Buzzword
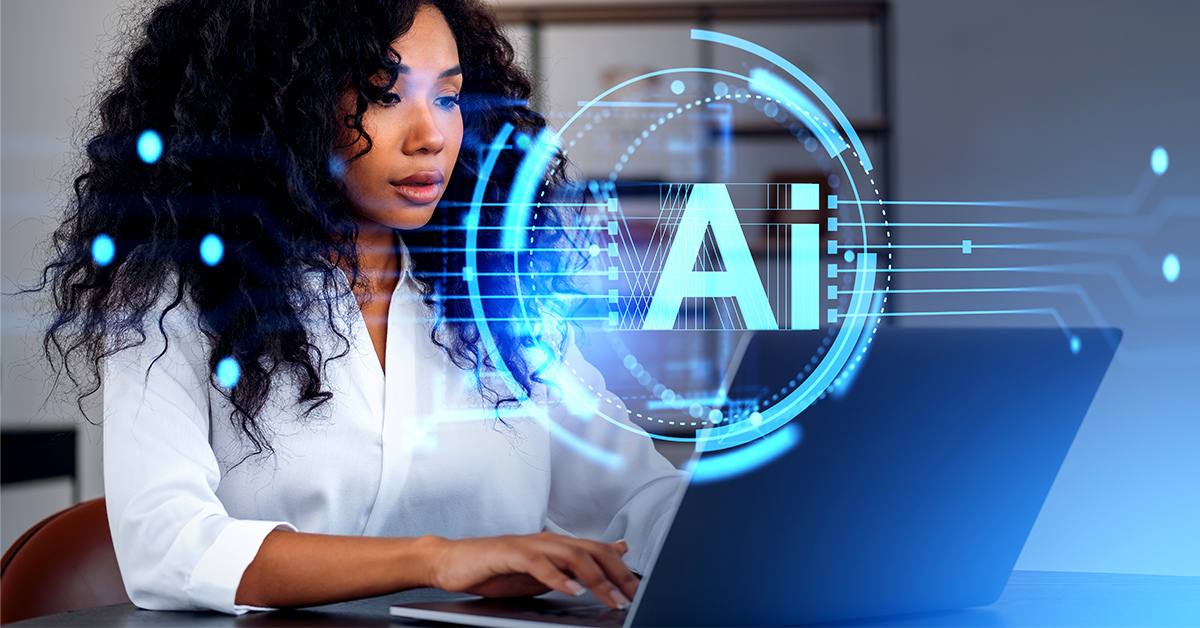
How AIOps Works: Continually Smarter & More Effective IT Operations
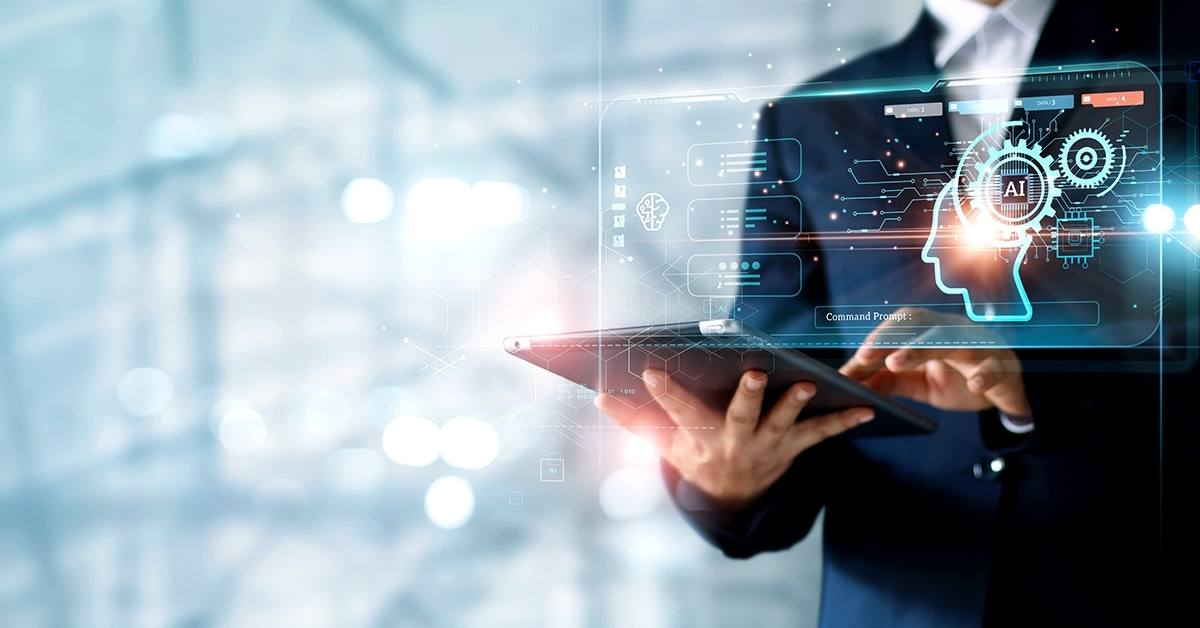
How AIOps Works: Tame Big Data and Get to the Crux of the Matter
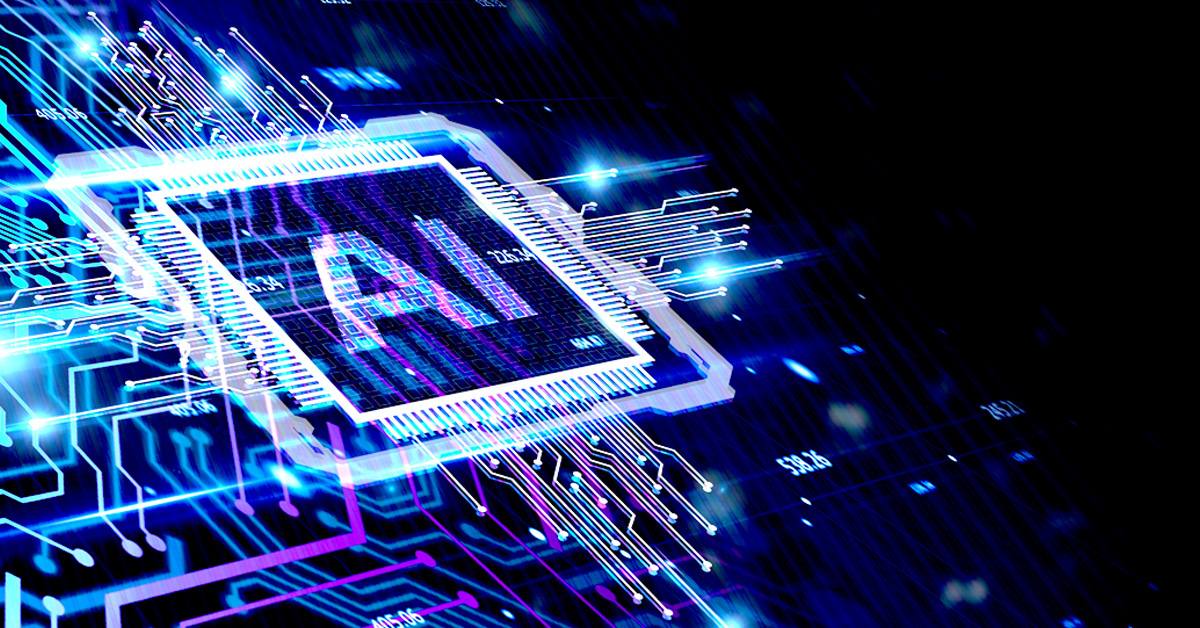
The Big Deal About AIOps
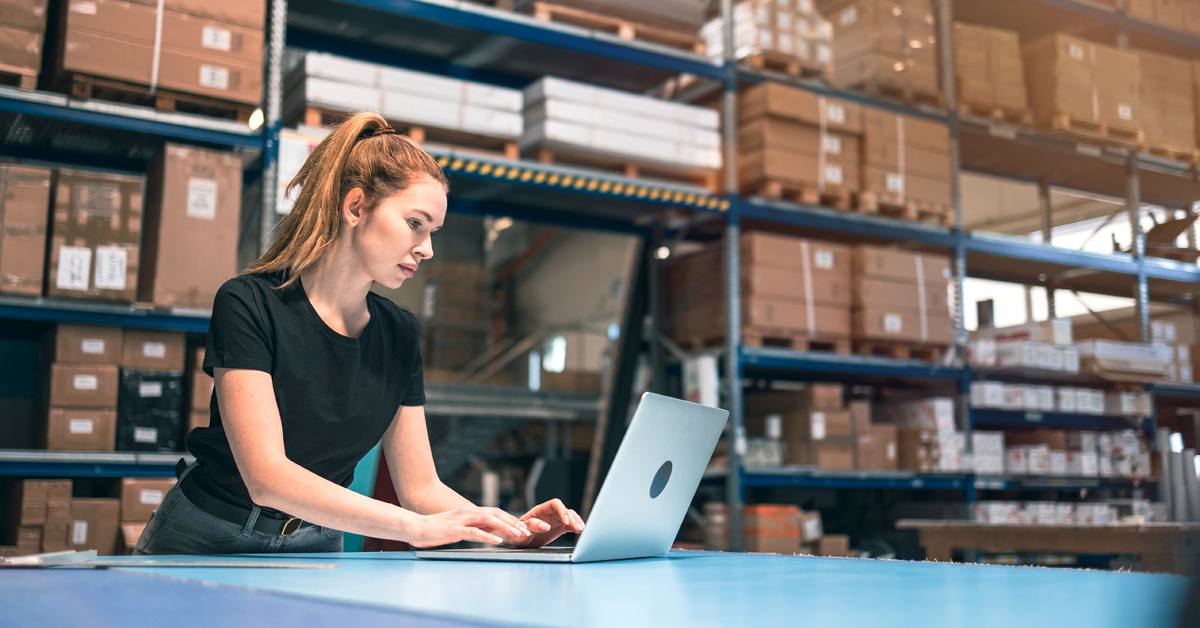
There’s More to IT Hardware Procurement Than Getting the Best Deal
CATEGORIES
The Big Deal About AIOps
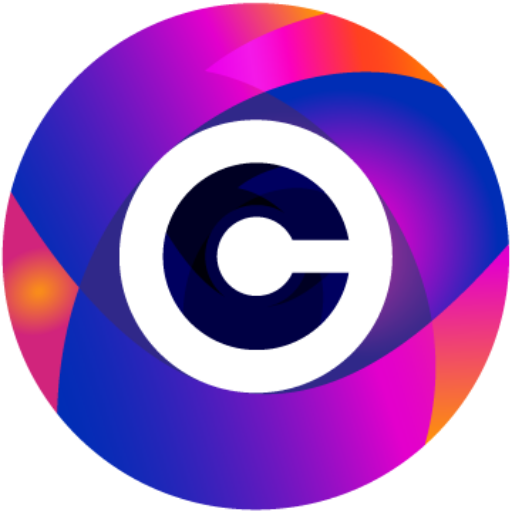
Greg Druffel • Managing Solution Architect
Why It’s the Future of IT Operations
How do you feel when you hear the term Artificial Intelligence (AI)? Excited? Anxious? Afraid? Suspicious? Were you an early adopter of ChatGPT, happily entering prompts to generate a week’s worth of Mediterranean diet recipes, or a blog that sounds like it Snoop Dogg or Cookie Monster wrote it? Or does the mention of AI make you shudder with thoughts of deep fakes and conspiracies, the voice of HAL 9000, SkyNet, and glitches in The Matrix? Frankly, for many of us, it’s a bit of both.
Whatever our trepidations, AI has rapidly moved from the realm of sci-fi to become part of our everyday lives. And the abundance of practical and beneficial applications for AI in business is undeniable – freeing us up from grunt work, augmenting our analytical capabilities, and saving us from costly human errors.
AIOps, or AI for IT Operations, is one such example. It uses Big Data, Machine Learning (ML), and AI to automate and improve how IT operations are managed, making them more efficient, reliable, and effective. This first blog in our five-part AIOps series gives an overview of AIOps and its benefits.
Cutting Through the Noise to Find and Fix the Issue
In enterprise organizations with complex and vast IT ecosystems, it’s becoming tougher to maintain a firm grip on IT operations. A typical enterprise organization has numerous applications, services, and infrastructure components in varied locations, generating huge amounts of data and requiring constant monitoring and analysis.
Pair this with increased user expectations and dependence on technology, and it’s easy to see the need for a system to help your IT teams cut through the noise to understand and predict which improvements are needed. And if this system can also automate some remedial actions, you’ll greatly augment your capability to keep your IT operations running smoothly and efficiently.
How Big Data and Machine Learning Work Together in AIOps
Big Data for AIOps refers to large, diverse sets of information sourced from monitoring systems and engagement data such as events, logs, performance, availability, and capacity.
ML is a subset of AI that focuses on algorithms that can learn from data and improve their performance without being explicitly programmed. In AIOps, ML can help discover patterns, trends, and correlations in the data, using them to make predictions and recommendations. It also enables AIOps to adapt to evolving environments and user needs by using feedback loops and reinforcement learning.
AIOps uses Big Data, AI, and ML capabilities to:
- Collect ever-increasing volumes of data from multiple IT infrastructure components.
- Intelligently pinpoint significant events and patterns related to performance and availability.
- Diagnose the root cause for automatic or human resolution.
The Components of an AIOps Implementation
According to a recent study, 67% of enterprise infrastructure is now cloud-based. A typical infrastructure can often be a combination of on-premises, private, and public cloud components and may be managed by multiple IT teams.
A successful AIOps implementation will carefully integrate several toolsets to help teams collaborate to observe, analyze, and act proactively to fix IT operations issues. These toolsets can be categorized into three functions:
- Data Ingestion
Observability tools handle the ingestion, aggregation, and analysis of performance data from your applications, infrastructure, and network. - Analysis, Correlation, and Recommendations
AIOps solutions analyze, correlate, and enrich the data for better insights and automated actions, enabling IT teams to filter out the noise from alerts and zero in on anomalies, patterns, and trends in resource requirements to get the big picture of your IT operations in real-time. - Proactive Remediation
AIOps tools can execute automated processes in response to recommendations, rectifying issues such as slowdowns promptly, sometimes before they even happen.
Get a Handle on Your IT Operations
Today’s companies face increased security threats and compliance requirements, difficulties managing vast quantities of system-generated data, and demands for complex and varied analysis by various stakeholders. Add to this list a shortage of IT talent to handle it all and it’s easy to see why there’s a dramatic rise in demand for AIOps.
As we continue with this blog series, we’ll dive deeper into how the components of AIOps work together, look at how an experienced provider like Compucom can help you go beyond the buzzword to realize its benefits and hear some real-world success stories.
In this series:
Recent Posts
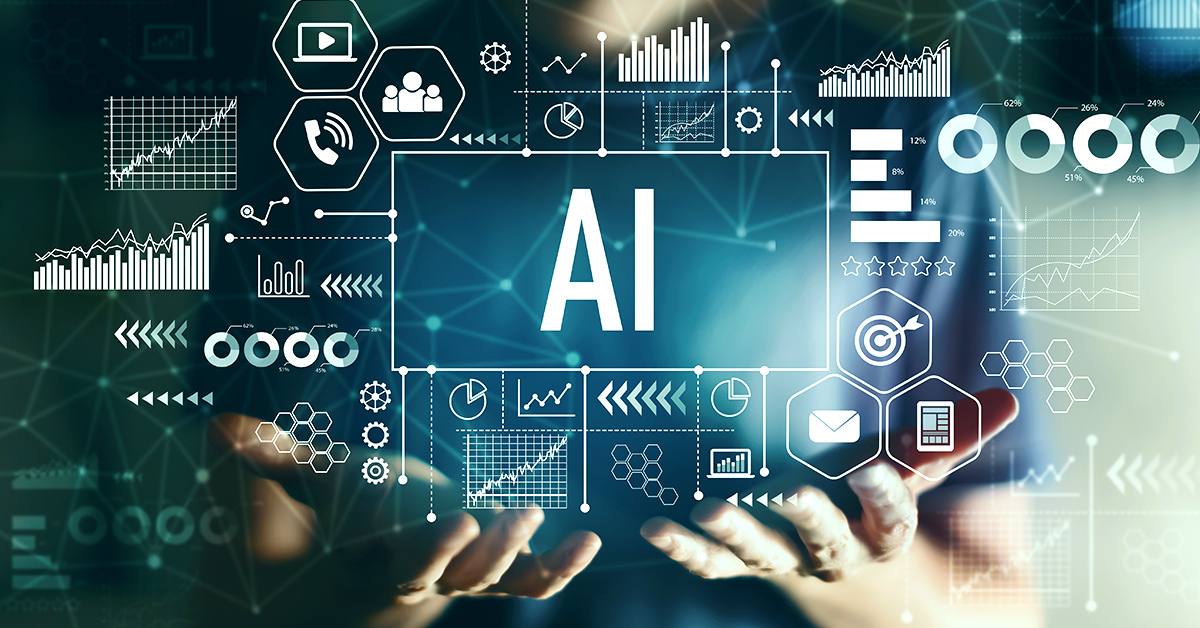
Real-World AIOps: Examples and Benefits
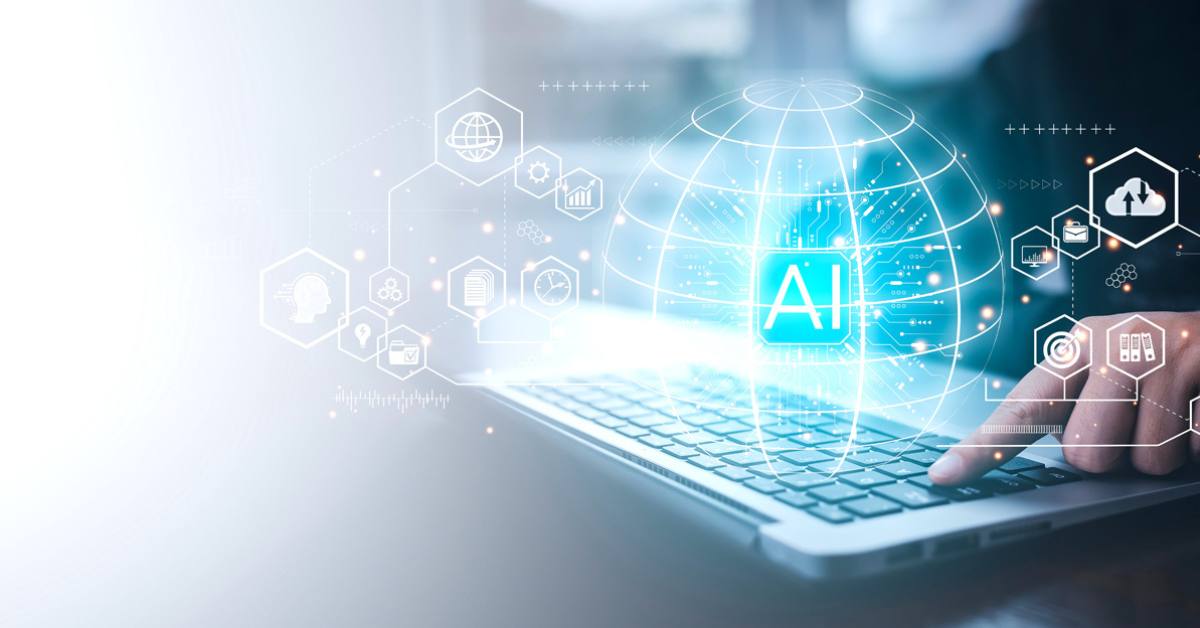
AIOps: Going Beyond the Buzzword
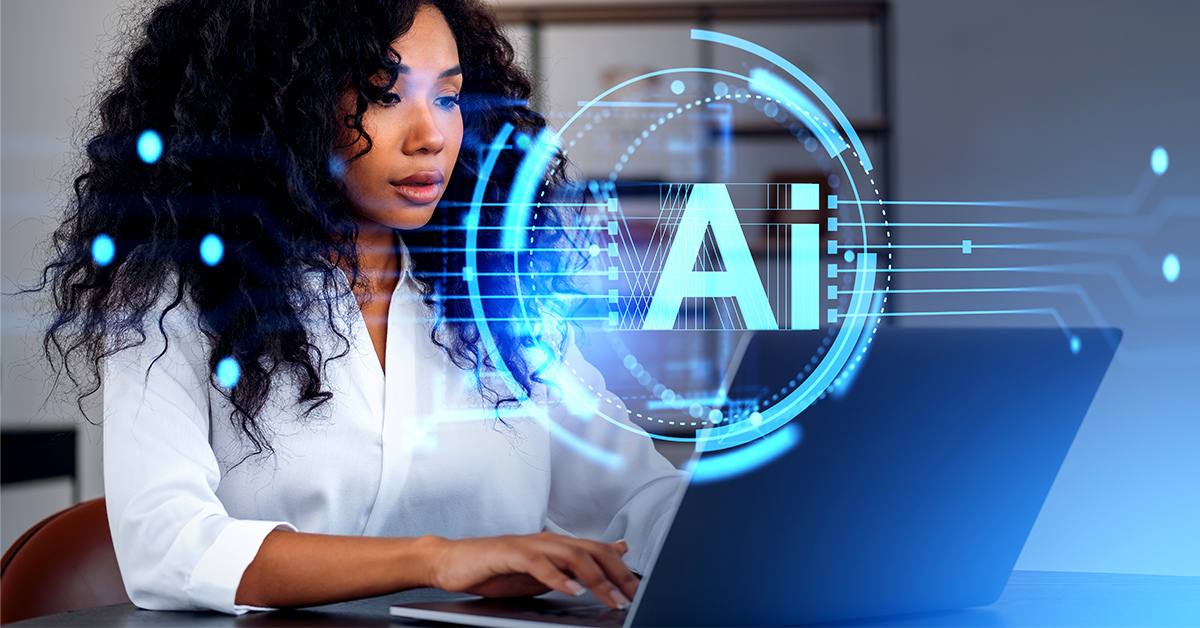
How AIOps Works: Continually Smarter & More Effective IT Operations
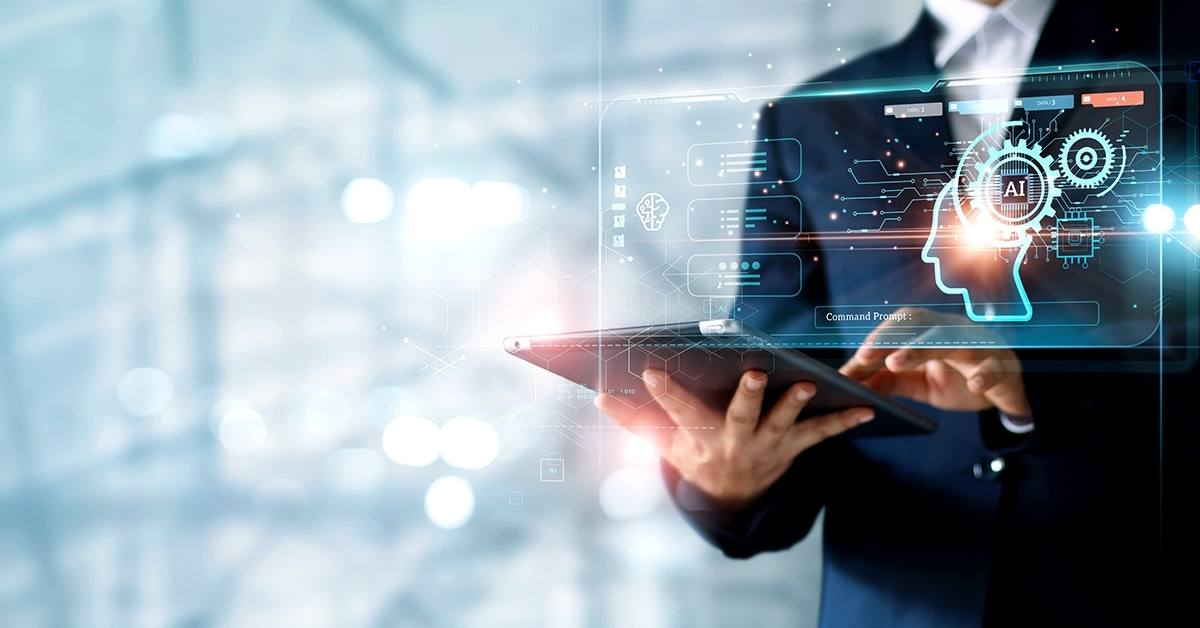
How AIOps Works: Tame Big Data and Get to the Crux of the Matter
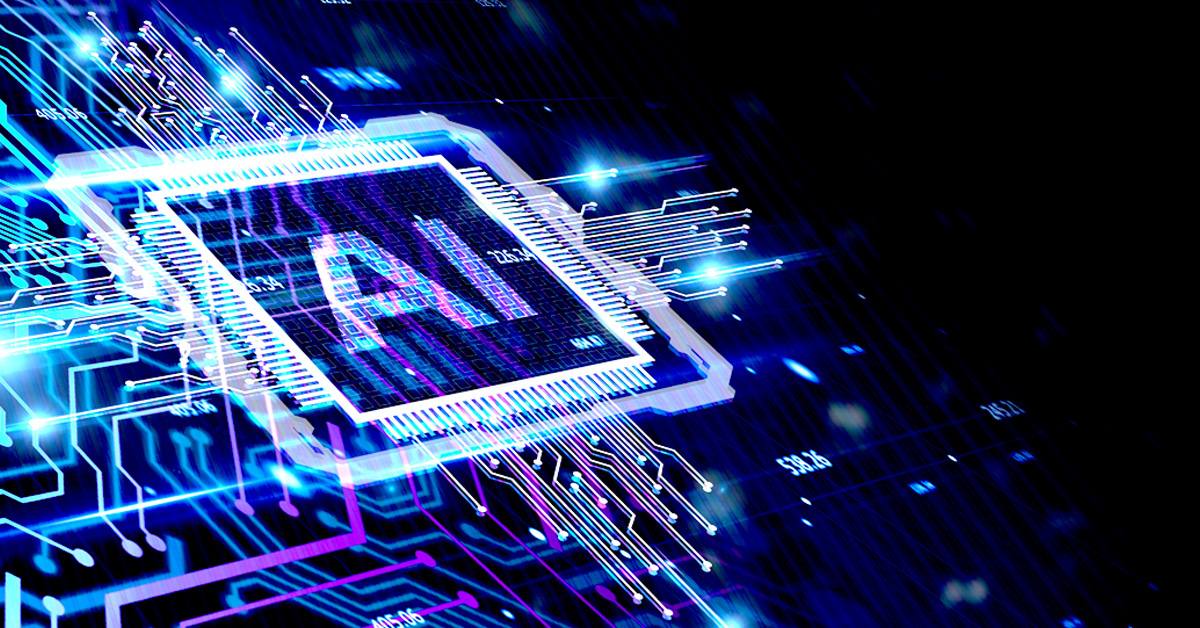
The Big Deal About AIOps
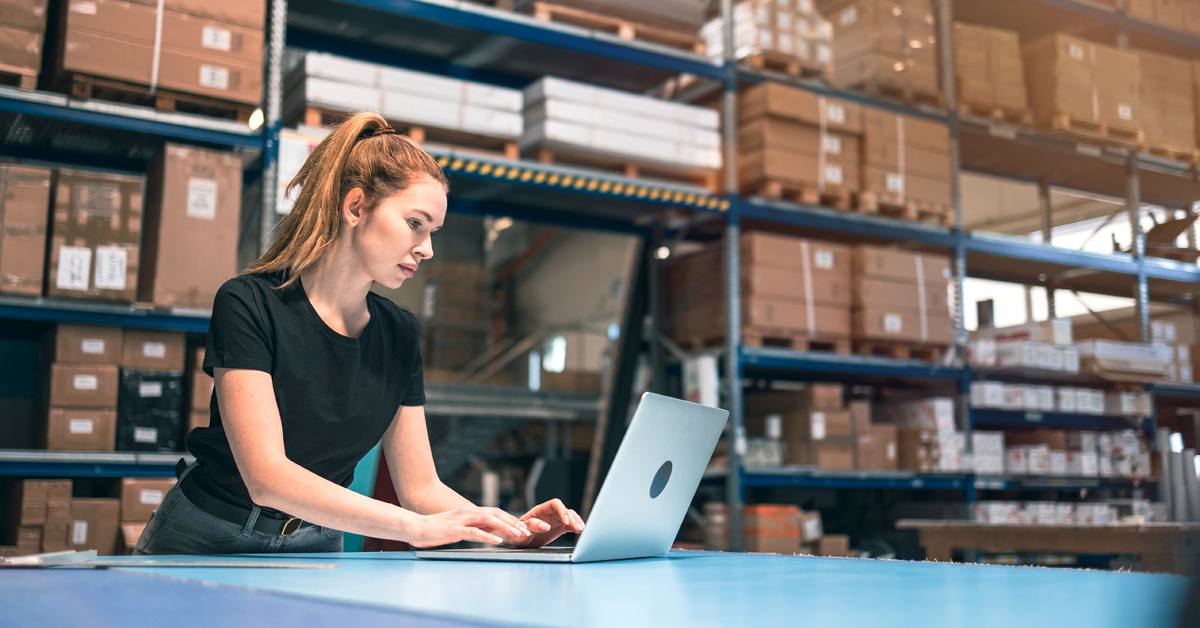
There’s More to IT Hardware Procurement Than Getting the Best Deal
CATEGORIES
Ready to Create an Innovative Workplace?
Recent Blog Posts
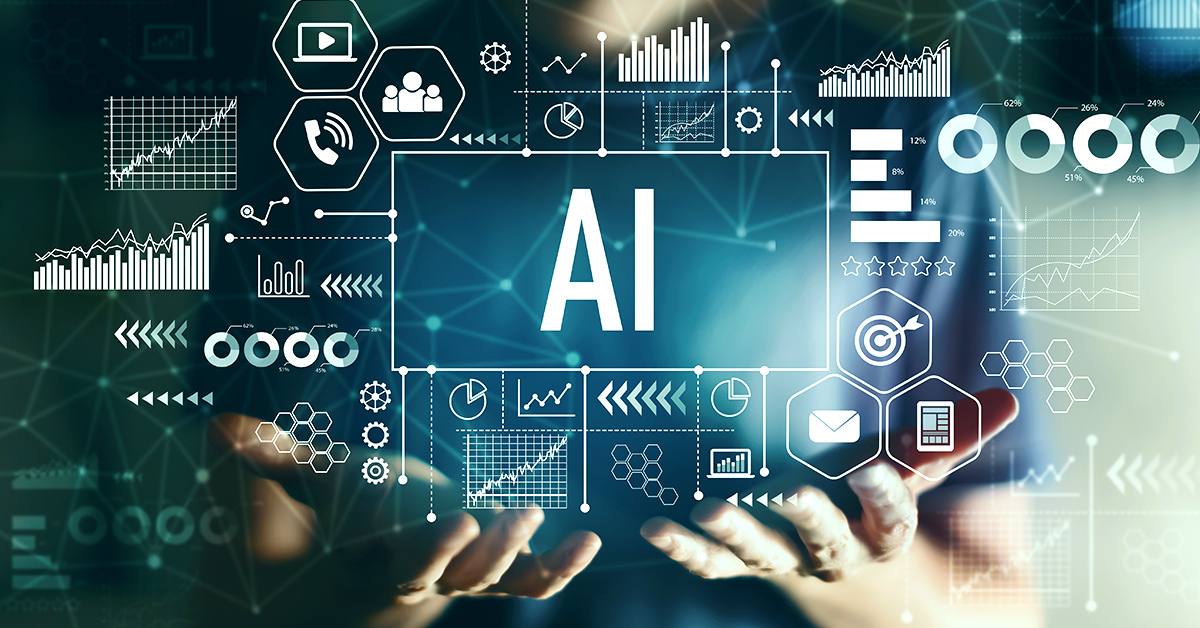
Real-World AIOps: Examples and Benefits
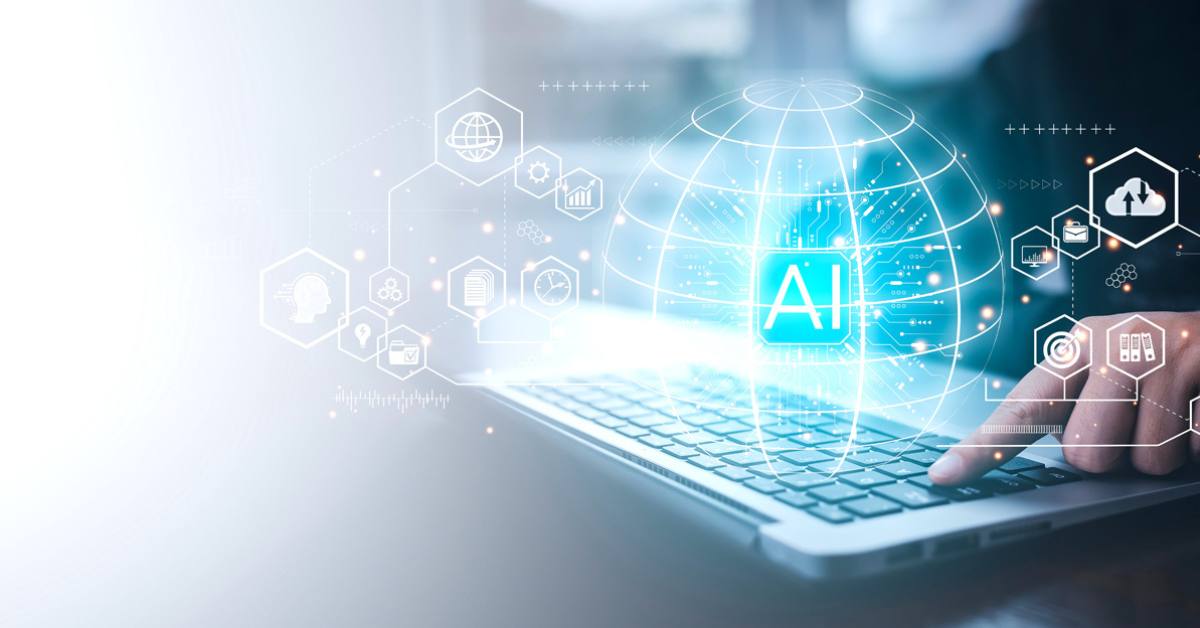
AIOps: Going Beyond the Buzzword
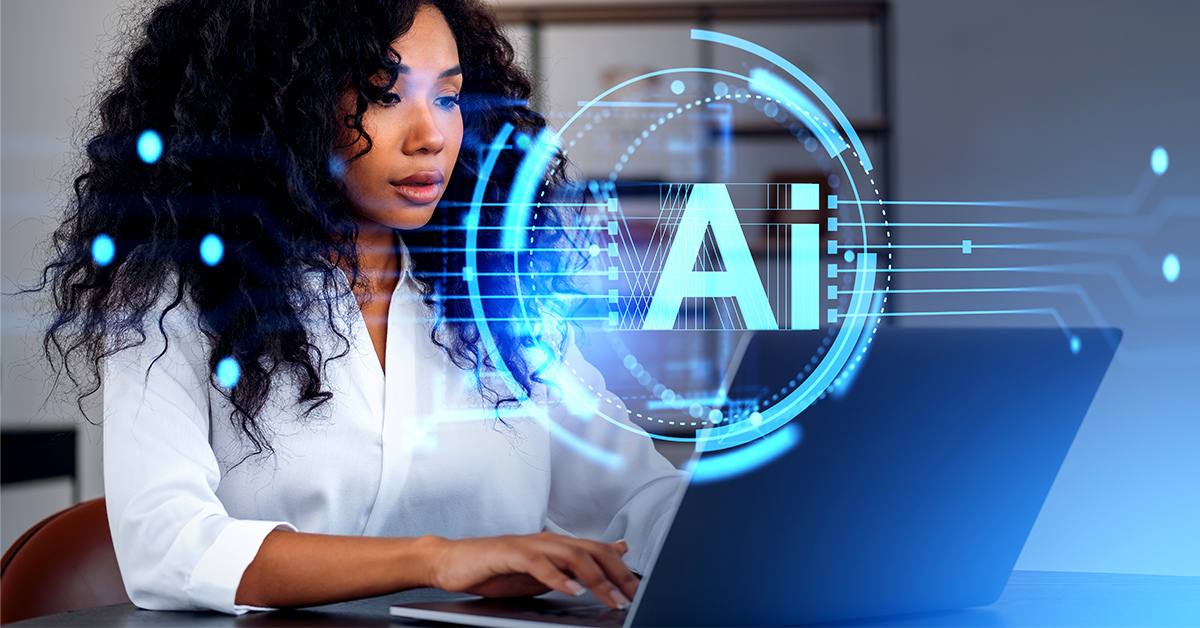